Executive Summary
The client is a manufacturer of heavy-duty vehicle surveillance systems. The company offers mobile video/back up camera, vehicle recording, fleet tracking, backing radar/object detection alarm, tire pressure monitoring, and event-triggered driver behavioral monitoring systems. The client was facing challenges such as delay in processing data and better reliability and accuracy of their driver assessment system. Hence, they were looking for a solution, which could help them in effective processing and analysis of drivers’ behavior and movement.
eInfochips proposed a solution combining the capability of Deep Learning with the existing driver assessment system. Our team of engineers trained a CNN (convolutional neural network) model with the real world data to develop the solution, which led to an increase in the accuracy of the driver assessment system. The system intelligently classifies, filters, and extracts various information related to driver behavior from the dash-cam videos that are captured when the driver is in the transit. It has the capabilities to cover a wide range of scenarios and road types, which further augments the capabilities of autonomous driving systems. Thus, it eliminates the manual monitoring and improves the accuracy of the whole system in real-world conditions.
Project Highlights
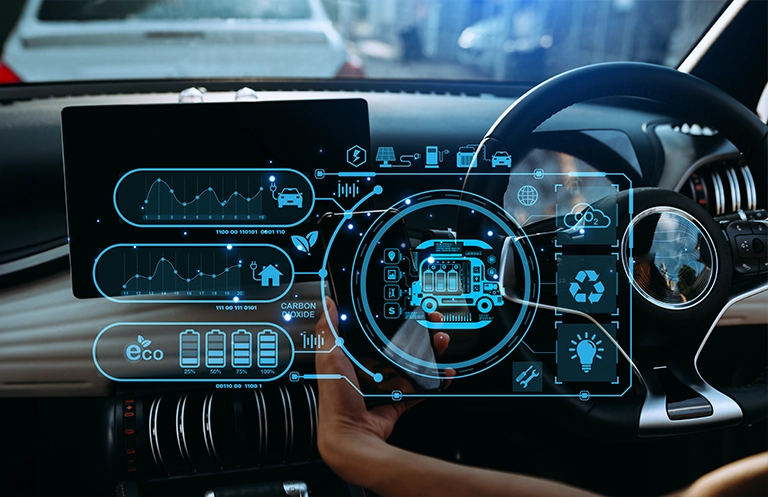
- CNN model trained on real-world data
- Driver image identification with 90% accuracy
- Driver yawning detection with 85% accuracy